Hit Identification
Hit identification is the pivotal first step in drug discovery, and at AI|ffinity, we leverage advanced techniques to identify promising ligands from vast chemical libraries. For novel targets, we employ traditional cheminformatics approaches such as docking and ligand-based screening, and are pioneering a hybrid ML-knowledge-based docking scoring function enhanced by our own pharmacophore fingerprints.
When dealing with targets with available binding information, we utilize our deep learning algorithm, deepHitMiner. This tool is a specialized adaptation of our award-winning deepScaffOpt algorithm (D3R Grand Challenges), specifically designed for large-scale virtual screening. Under appropriate circumstances, such as the presence of sufficient binding data for analogous proteins, deepHitMiner can be applied to new targets through "transfer learning". This process enables deepHitMiner to generate a classifier model that predicts the likelihood of a new compound binding to our target protein. Given even a small number of compounds with known binding information for our target, this model can be finely tuned for enhanced performance. Additionally, when experimental data from 1D NMR screening is available, both deepHitMiner and deepScaffOpt can be optimized for improved predictive accuracy.
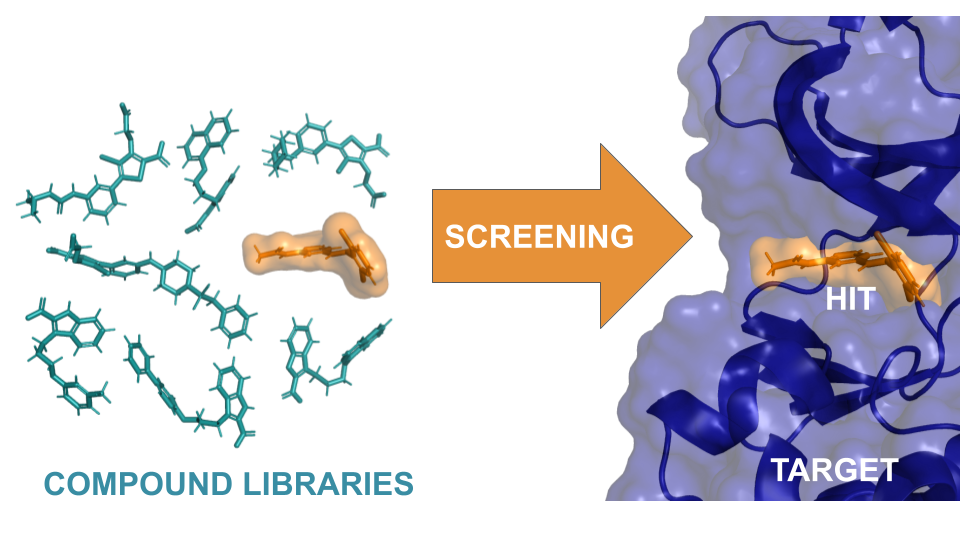
deepHitMiner’s Special Features:
- Independence from Receptor 3D Structure: Beneficial but not necessary.
- Diverse Molecular Representations: Over 60 types catering to varied drug design requirements.
- Exclusive 1D NMR Enhancement: Enhances 2D molecular representations using NMR screening data. An industry-exclusive feature.
- Tailored Deep Learning Approach: Includes custom neural network layers, a unique loss function, and an early stopping method for precise binding predictions.
- Enhanced Learning with Transfer Learning: Utilizes binding data from related targets to improve hit discovery rates.
- Advanced Multi-input Neural Networks: Combines multiple molecular representations for bespoke receptor and library solutions, maximizing prediction accuracy.
- Streamlined Installation: Packaged within a docker image with all required dependencies for a hassle-free one-click setup.